BST 236: Computing I
Course Website [Link]
Syllabus
This course is a beginner-friendly course on statistical computing in the era of generative AI. We are going to cover the topics in:
- Principles of good coding practices, code efficiency and reproducibility, Git and GitHub version control, Makefile automation, virtual environments
- AI copilot integration, prompt engineering, AI-assisted coding
- Python built-in data structures (lists, dictionaries, deque), NumPy arrays and operations, Pandas DataFrames and data manipulation
- Computational complexity analysis, memory complexity, recursion techniques, backtracking algorithms, dynamic programming, greedy algorithms
- Algorithms for solving linear equations, eigenvalue decomposition, singular value decomposition, matrix factorizations
- Convex optimization methods, stochastic optimization algorithms, non-convex optimization techniques, gradient-based methods
- PyTorch framework, neural network architectures, transformer models, deep learning training and optimization
BST 235: Advanced Regression and Statistical Learning
Lecture Notes [PDF]
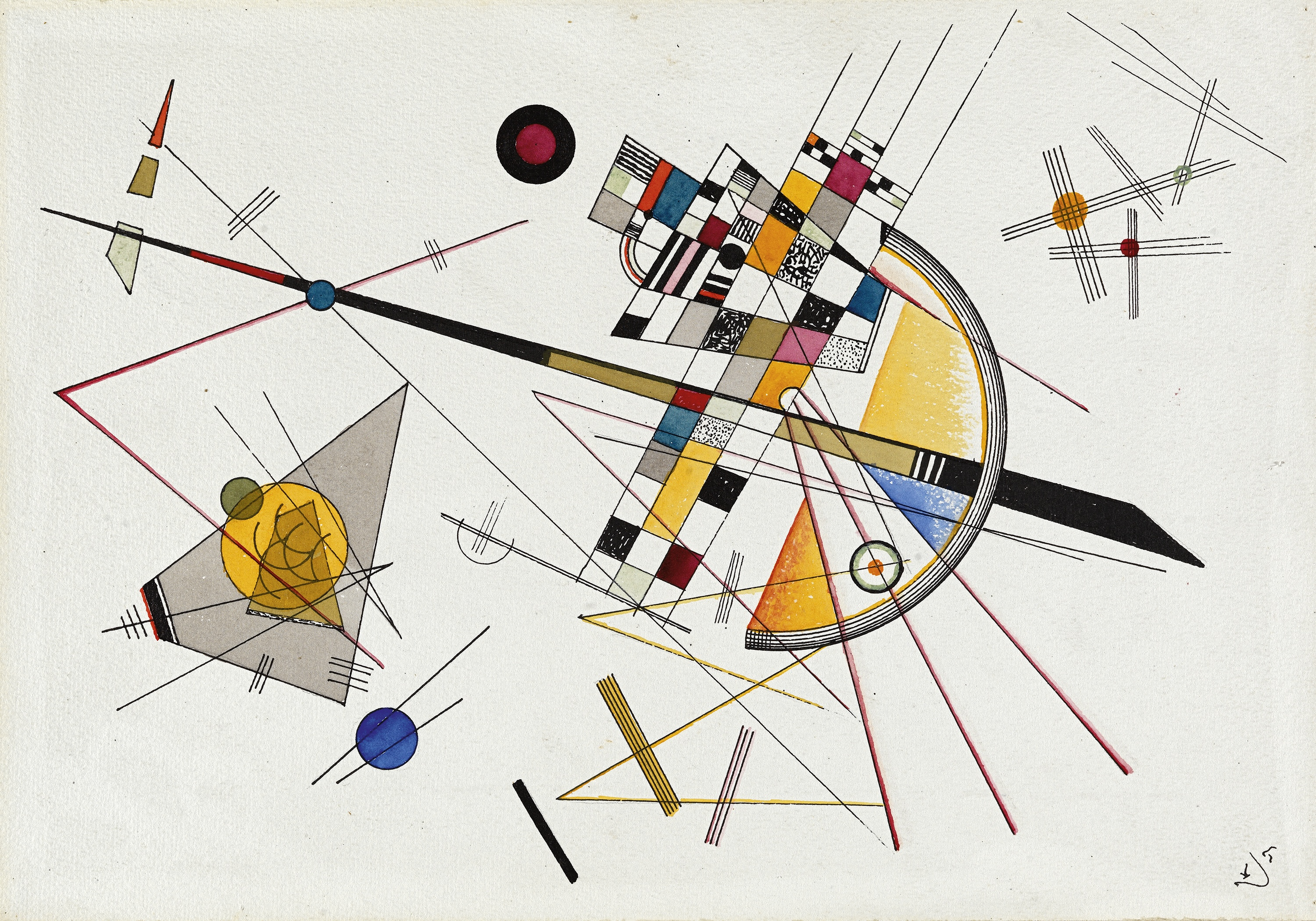
Syllabus
This course is an advanced course on the methods and theory of high dimensional statistics, statistical machine learning, and large-scale inference and optimization. We aim to quickly bring students to the frontier and interdisciplinary areas of statistics, optimization, probability, and machine learning. We are going to cover the topics in:
- Concentration inequality, Sub-Gaussian random variables, Chernoff bounds, Hoeffding's inequality, Maximal inequalities
- Ordinary least square, Compressed sensing, Lasso, Variations of Lasso including group lasso, fused lasso, adaptive lasso, etc., General high dimensional M- estimators, Variable selection consistency
- Convex geometry, Lagrange duality, Gradient descent, Proximal gradient descent, LARS, ADMM, Mirror descent, Stochastic optimization
- Linear model hypothesis testing, high dimensional inference, Chi-square test, maximal test, False discovery rate control, Knockoff filter
BST 263: Statistical Learning
Lecture Notes [PDF]
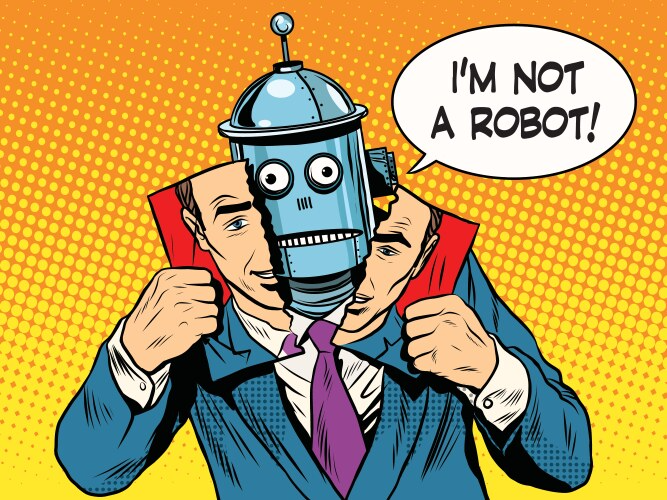
Syllabus
This course introduces statistical machine learning under the big umbrella of data, sincere and modern analytics. More detailed topics include:
- OLS, Lasso, Ridge, Elastic-Net, Tree based methods, Random Forests
- Logistic regression, Support vector machine, Linear discriminant analysis, Naive Bayes, Boosting
- Mixture of Gaussians, Expectation maximization algorithm, K-means
- Deep learning, Reinforcement learning